Python is a popular tool for implementing web scraping. Python programming language is also used for other useful projects related to cyber security, penetration testing as well as digital forensic applications. Using the base programming of Python, web scraping can be performed without using any other third party tool. Python programming. Storing the results of Web Scraping into Database. Ask Question Asked 2 years, 5 months ago. Active 2 years, 5 months ago. Viewed 3k times 5. I have written a code for web scraping using python. The code extracts data of Macbook from amazon using selenium.
I thought, how can we angle 'Web Scraping for Machine Learning', and I realized that Web Scraping should be essential to Data Scientists, Data Engineers and Machine Learning Engineers.
The Full Stack AI/ML Engineer toolkit needs to include web scraping, because it can improve predictions with new quality data. Machine Learning inherently requires data, and we would be most comfortable, if we have as much high quality data as possible. But what about when the data you need is not available as a dataset? What then? Do you just go and ask organizations and hope that they kindly will deliver it to you for free?
The answer is: you collect, label and store it yourself.
I made a GitHub repository for scraping the data. I encourage you to try it out and scrape some data yourself, and even trying to make some NLP or other Machine Learning project out of the scraped data.
In this article, we are going to web scrape Reddit – specifically, the /r/DataScience (and a little of /r/MachineLearning) subreddit. There will be no usage of the Reddit API, since we usually web scrape when an API is not available. Furthermore, you are going to learn to combine the knowledge of HTML, Python, Databases, SQL and datasets for Machine Learning. We are doing a small NLP sample project at last, but this is only to showcase that you can pickup the dataset and create a model providing predictions.
- Web Scraping in Python - Beautiful Soup and Selenium
- Extracting Data From Python Dict
- Labelling Scraped Data
- Storing Scraped Data
Web Scraping in Python With BeautifulSoup and Selenium
The first things we need to do is install BeautifulSoup and Selenium for scraping, but for accessing the whole project (i.e. also the Machine Learning part), we need more packages.
Selenium is basically good for content that changes due to Javascript, while BeautifulSoup is great at capturing static HTML from pages. Both the packages can be downloaded to your environment by a simple pip or conda command.
Install all the packages from the Github (linked at the start)
Alternatively, if you are using Google Colab, you can run the following to install the packages needed:
Next, you need to download the chromedriver and place it in the core folder of the downloaded repository from GitHub.
Basic Warning of Web Scraping
Scrape responsibly, please! Reddit might update their website and invalidate the current approach of scraping the data from the website. If this is used in production, you would really want to setup an email / sms service, such that you get immediate notice when your web scraper fails.
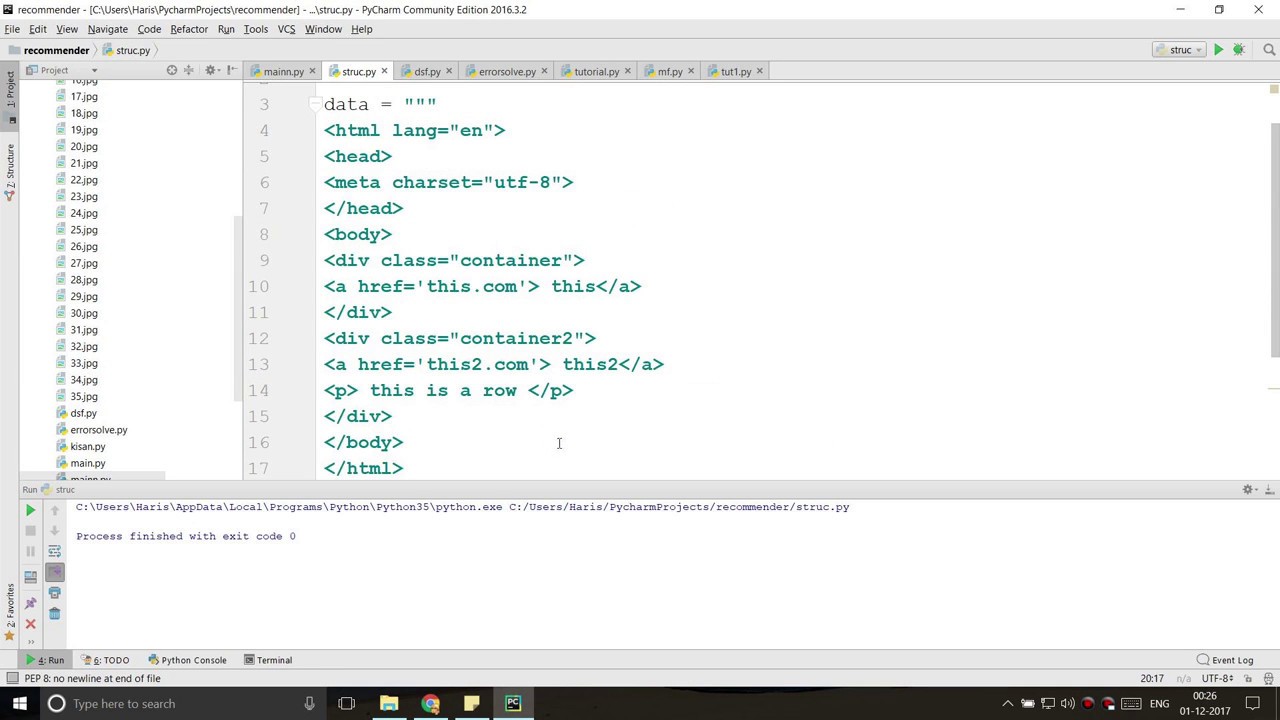
This is for educational purposes only, please don't misuse or do anything illegal with the code. It's provided as-is, by the MIT license of the GitHub repository.
Benchmarking How Long It Takes To Scrape
Scraping takes time. Remember that you have to open each page, letting it load, then scraping the needed data. It can really be a tedious process – even figuring out where to start gathering the data can be hard, or even figuring out exactly what data you want.
There are 5 main steps for scraping reddit data:
- Collecting links from a subreddit
- Finding the <script> tag from each link
- Turning collected data from links into Python dictionaries
- Getting the data from Python dictionaries
- Scraping comment data
Especially step 2 and 5 will take you a long time, because they are the hardest to optimize.
An approximate benchmark for:
- Step 2: about 1 second for each post
- Step 5: $n/3$ seconds for each post, where $n$ is number of comments. Scraping 300 comments took about 100 seconds for me, but it varies with internet speed, CPU speed, etc.
Initializing Classes From The Project
We are going to be using 4 files with one class each, a total of 4 classes, which I placed in the core folder.
Whenever you see SQL, SelScraper or BSS being called, it means we are calling a method from another class, e.g. BSS.get_title()
. We are going to jump forward and over some lines of code, because about 1000 lines have been written (which is too much to explain here).
Scraping URLs From A Subreddit
We start off with scraping the actual URLs from a given subreddit, defined at the start of scraper.py
. What happens is that we open a browser in headless mode (without opening a window, basically running in the background), and we use some Javascript to scroll the page, while collecting links to posts.
The next snippets are just running in a while loop until the variable scroll_n_times
becomes zero
The following is the Javascript to scroll a page to the bottom:
After that, we use xpath to find all <a> tags in the HTML body, which really just returns all the links to us:
At any point in time, if some exception happens, or we are done with scrolling, we basically garbage collect the process running, such that the program won't have 14 different chrome browsers running
Great, now we have a collection of links that we can start scraping, but how? Well, we start by...
Getting the data attribute
Upon opening one of the collected links, Reddit provides us with a javascript <script> element that contains all the data for each post. You won't be able to see it when visiting the page, since the data is loaded in, then removed.
But our great software package BeautifulSoup will! And for our convenience, Reddit marked the <script> with an id: id=data
. This attribute makes it easy for us to find the element and capture all the data of the page.
First we specify the header, which tells the website we are visiting, which agent we are using (as to avoid being detected as a bot). Next step, we make a request and let BeautifulSoup get all the text from the page, i.e. all the HTML.
You see that last line, after we have told BeautifulSoup to load the page, we find the text of the script with the id='data'
. Since it's an id attribute, I knew that there would only be one element on the whole page with this attribute – hence this is pretty much a bulletproof approach of getting the data. Unless, of course, Reddit changes their site.
You might think that we could parallelize these operations, such that we can run through multiple URLs in the for loop, but it's bad practice and might get you banned – especially on bigger sites, they will protect their servers from overload, which you effectively can do with pinging URLs.
Converting the <script> string to Python dictionary

From the last code piece, we get a string, which is in a valid JSON format. We want to convert this string into a Python dict, such that we can easily lookup the data from each link.
The basic approach here is that we find the first left curly bracket, which is where JSON starts, by the index. And we find the last index by an rfind() method with the right curly bracket, but we need to use plus one for the actual index.
Effectively, this gives us a Python dict of the whole Reddit data which is loaded into every post. This will be great for scraping all the data and storing it.
Actually Getting The Data (From Python dictionary)
I defined one for loop, which iteratively scrapes different data. This makes it easy to maintain and find your mistakes.
As you can see, we get quite a lot of data – basically the whole post and comments, except for comments text, which we get later on.
Scraping Comments Text Data
After we collected all this data, we need to begin thinking about storing the data. But we couldn't manage to scrape the text of the comments in the big for loop, so we will have to do that before we setup the inserts into the database tables.
This next code piece is quite long, but it's all you need. Here we collect comment text, score, author, upvote points and depth. For each comment, we have subcomments for that main comment, which specifies depth, e.g. depth is zero at the each root comment.
What I found working was opening each of the collected comment URLs from the earlier for loop, and basically scraping once again. This ensures that we get all the data scraped.
Note: this approach for scraping the comments can be reaaally slow! This is probably the number one thing to improve – it's completely dependent on the number of comments on a posts. Scraping >100 comments takes a pretty long time.
Labelling The Collected Data
For the labelling part, we are mostly going to focus on tasks we can immediately finish with Python code, instead of the tasks that we cannot. For instance, labelling images found on Reddit is probably not feasible by a script, but actually has to be done manually.
Naming Conventions
For the SQL in this article, we use Snake Case for naming the features. An example of this naming convention is my_feature_x, i.e. we split words with underscores, and only lower case.
...But please:
If you are working in a company, look at the naming conventions they use and follow them. The last thing you want is different styles, as it will just be confusing at the end of the day. Common naming conventions include camelCase and Pascal Case.
Storing The Labelled Data
For storing the collected and labelled data, I have specifically chosen that we should proceed with an SQLite database – since it's way easier for smaller projects like web scraping. We don't have to install any drivers like for MySQL or MS SQL servers, and we don't even need to install any packages to use it, because it comes natively with Python.
Some considerations for data types has been made for the columns in the SQLite database, but there is room for improvement in the current state of form. I used some cheap varchars in the comment table, to get around some storing problems. It currently does not give me any problems, but for the future, it should probably be updated.
Designing and Creating Tables
The 1st normal form was mostly considered in the database design, i.e. we have separate tables for links and comments, for avoiding duplicate rows in the post table. Further improvements include making a table for categories and flairs, which is currently put into a string form from an array.
Without further notice, let me present you the database diagram for this project. It's quite simple.
In short: we have 3 tables. For each row with a post id in the post table, we can have multiple rows with the same post id in the comment and link table. This is how we link the post to all links and comments. The link exists because the post_id in the link and comment table has a foreign key on the post_id in the post table, which is the primary key.
I used an excellent tool for generating this database diagram, which I want to highlight – currently it's free and it's called https://dbdiagram.io/.
Play around with it, if you wish. This is not a paid endorsement of any sorts, just a shoutout to a great, free tool. Here is my code for the above diagram:
Actually Inserting The Data
The databases and tables will be automatically generated by some code which I setup to run automatically, so we will not cover that part here, but rather, the part where we insert the actual data.
The first step is creating and/or connecting to the database (which will either automatically generate the database and tables, and/or just connect to the existing database).
After that, we begin inserting data.
Inserting the data happens in a for loop, as can be seen from the code snippet above. We specify the column and the data which we want to input.
For the next step, we need to get the number of columns in the table we are inserting into. From the number of columns, we have to create an array of question marks – we have one question mark separated with a comma, for each column. This is how data is inserted, by the SQL syntax.
Some data is input into a function which I called insert(), and the data variable is an array in the form of a row. Basically, we already concatenated all the data into an array and now we are ready to insert.
This wraps up scraping and inserting into a database, but how about...
Exporting From SQL Databases
For this project, I made three datasets, one of which I used for a Machine Learning project in the next section of this article.
- A dataset with posts, comments and links data
- A dataset with the post only
- A dataset with comments only
For these three datasets, I made a Python file called make_dataset.py
for creating the datasets and saving them using Pandas and some SQL query.
For the first dataset, we used a left join from the SQL syntax (which I won't go into detail about), and it provides the dataset that we wish for. You do have to filter a lot of nulls if you want to use this dataset for anything, i.e. a lot of data cleaning, but once that's done, you can use the whole dataset.
For the second and third datasets, a simple select all from table SQL query was made to make the dataset. This needs no further explaining.
Machine Learning Project Based On This Dataset
From the three generated datasets, I wanted to show you how to do a basic machine learning project.
The results are not amazing, but we are trying to classify the comment into four categories; exceptional, good, average and bad – all based on the upvotes on a comment.
Let's start! Firstly, we import the functions and packages we need, along with the dataset, which is the comments table. But we only import the score (upvotes) and comment text from that dataset.
The next thing we have to do is cleaning the dataset. Firstly, we start off with only getting the text by using some regular expression (regex). This removes any weird characters like | / & % etc.
The next thing we do is with regards to the score feature. The score feature is formatted as a string, and we just need the number from that string. But we also want the minus in front of the string, if some comment has been downvoted a lot. Another regex was used here to do this.
The next ugly thing from our Python script is a None as a string. We replace this string with an actual None in Python, such that we can run df.dropna().
The last thing we need to do is convert the score to a float, since that is required for later.
The next part is trying to define how our classification is going to work; so we have to adapt the data. An easy way is using percentiles (perhaps not an ideal way).
For this, we find the fiftieth, seventy-fifth and ninety-fifth quantile of the data and mark the data below the fiftieth quantile. We replace the score feature with this new feature.
We need to split the data and tokenize the text, which we proceed to do in the following code snippet. There is really not much magic happening here, so let's move on.
The last part of this machine learning project tutorial is making predictions and scoring the algorithm we choose to go with.
Logistic Regression was used, and perhaps we did not get the best score, but this is merely a boilerplate for future improvement and use.
All we do here is fit the logistic regression model to the training data, make a prediction and then score how well the model predicted.
In our case, the predictions were not that great, as the accuracy turned out to be $0.59$.
Future works includes:
- Fine-tuning different algorithms
- Trying different metrics
- Better data cleaning
Further Readings
For this last section, I want to link to some important documentation for web scraping and SQL in Python.
What is Web Scraping?
Web Scraping is a technique to extract a large amount of data from several websites. The term 'scraping' refers to obtaining the information from another source (webpages) and saving it into a local file. For example: Suppose you are working on a project called 'Phone comparing website,' where you require the price of mobile phones, ratings, and model names to make comparisons between the different mobile phones. If you collect these details by checking various sites, it will take much time. In that case, web scrapping plays an important role where by writing a few lines of code you can get the desired results.
Web Scrapping extracts the data from websites in the unstructured format. It helps to collect these unstructured data and convert it in a structured form.
Startups prefer web scrapping because it is a cheap and effective way to get a large amount of data without any partnership with the data selling company.
Is Web Scrapping legal?
Here the question arises whether the web scrapping is legal or not. The answer is that some sites allow it when used legally. Web scraping is just a tool you can use it in the right way or wrong way.
Web scrapping is illegal if someone tries to scrap the nonpublic data. Nonpublic data is not reachable to everyone; if you try to extract such data then it is a violation of the legal term.
There are several tools available to scrap data from websites, such as:
- Scrapping-bot
- Scrapper API
- Octoparse
- Import.io
- Webhose.io
- Dexi.io
- Outwit
- Diffbot
- Content Grabber
- Mozenda
- Web Scrapper Chrome Extension
Why Web Scrapping?
As we have discussed above, web scrapping is used to extract the data from websites. But we should know how to use that raw data. That raw data can be used in various fields. Let's have a look at the usage of web scrapping:
- Dynamic Price Monitoring
It is widely used to collect data from several online shopping sites and compare the prices of products and make profitable pricing decisions. Price monitoring using web scrapped data gives the ability to the companies to know the market condition and facilitate dynamic pricing. It ensures the companies they always outrank others.
- Market Research
eb Scrapping is perfectly appropriate for market trend analysis. It is gaining insights into a particular market. The large organization requires a great deal of data, and web scrapping provides the data with a guaranteed level of reliability and accuracy.
- Email Gathering
Many companies use personals e-mail data for email marketing. They can target the specific audience for their marketing.
- News and Content Monitoring
A single news cycle can create an outstanding effect or a genuine threat to your business. If your company depends on the news analysis of an organization, it frequently appears in the news. So web scraping provides the ultimate solution to monitoring and parsing the most critical stories. News articles and social media platform can directly influence the stock market.
- Social Media Scrapping
Web Scrapping plays an essential role in extracting data from social media websites such as Twitter, Facebook, and Instagram, to find the trending topics.
- Research and Development
The large set of data such as general information, statistics, and temperature is scrapped from websites, which is analyzed and used to carry out surveys or research and development.
Why use Python for Web Scrapping?
There are other popular programming languages, but why we choose the Python over other programming languages for web scraping? Below we are describing a list of Python's features that make the most useful programming language for web scrapping.
- Dynamically Typed
In Python, we don't need to define data types for variables; we can directly use the variable wherever it requires. It saves time and makes a task faster. Python defines its classes to identify the data type of variable.
- Vast collection of libraries
Python comes with an extensive range of libraries such as NumPy, Matplotlib, Pandas, Scipy, etc., that provide flexibility to work with various purposes. It is suited for almost every emerging field and also for web scrapping for extracting data and do manipulation.
- Less Code
The purpose of the web scrapping is to save time. But what if you spend more time in writing the code? That's why we use Python, as it can perform a task in a few lines of code.
- Open-Source Community
Python is open-source, which means it is freely available for everyone. It has one of the biggest communities across the world where you can seek help if you get stuck anywhere in Python code.
The basics of web scraping
The web scrapping consists of two parts: a web crawler and a web scraper. In simple words, the web crawler is a horse, and the scrapper is the chariot. The crawler leads the scrapper and extracts the requested data. Let's understand about these two components of web scrapping:
- The crawler
A web crawler is generally called a 'spider.' It is an artificial intelligence technology that browses the internet to index and searches for the content by given links. It searches for the relevant information asked by the programmer.
A web scraper is a dedicated tool that is designed to extract the data from several websites quickly and effectively. Web scrappers vary widely in design and complexity, depending on the projects.
How does Web Scrapping work?
These are the following steps to perform web scraping. Let's understand the working of web scraping.
Step -1: Find the URL that you want to scrape
First, you should understand the requirement of data according to your project. A webpage or website contains a large amount of information. That's why scrap only relevant information. In simple words, the developer should be familiar with the data requirement.
Step - 2: Inspecting the Page
The data is extracted in raw HTML format, which must be carefully parsed and reduce the noise from the raw data. In some cases, data can be simple as name and address or as complex as high dimensional weather and stock market data.
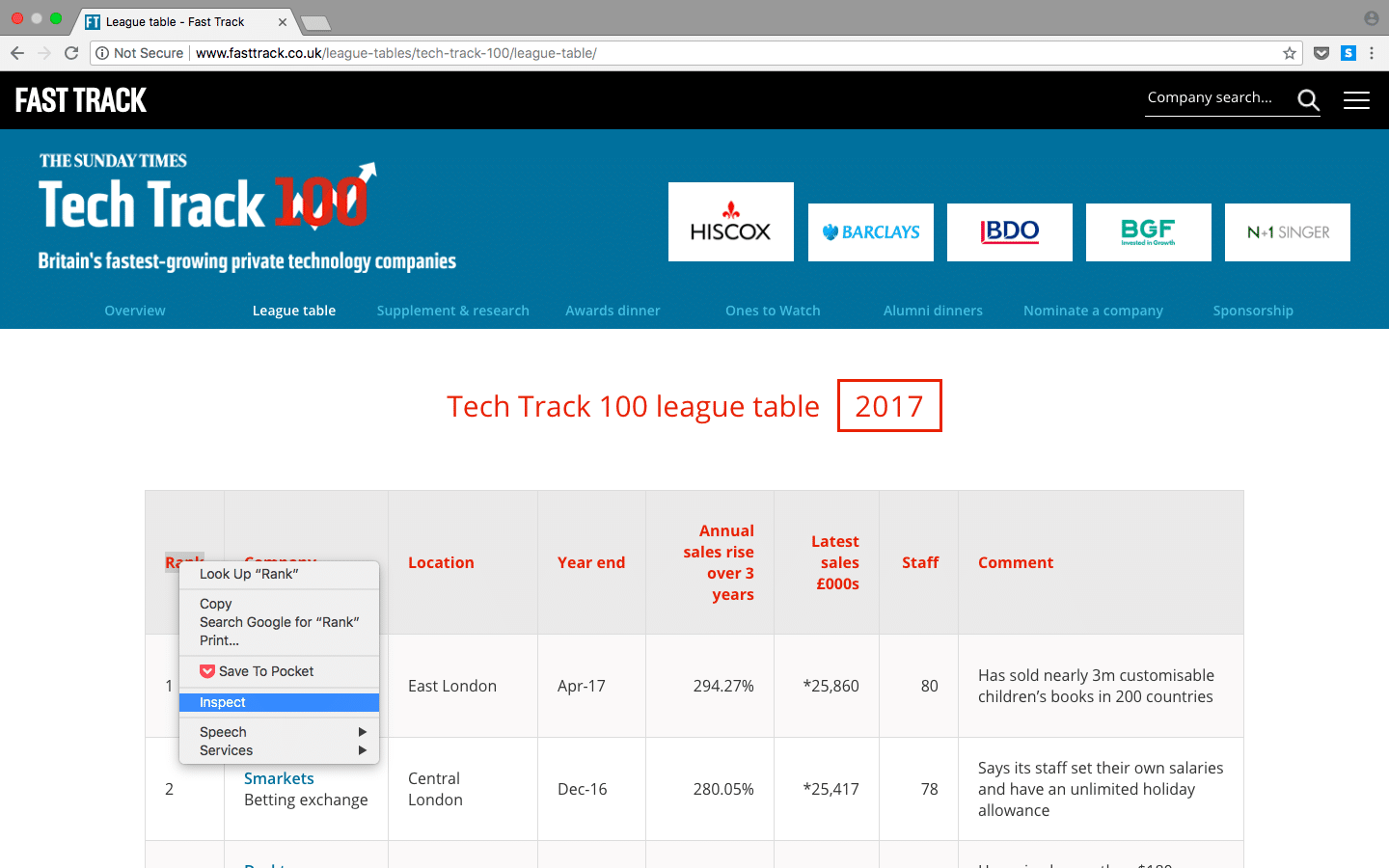
Step - 3: Write the code
Write a code to extract the information, provide relevant information, and run the code.
Step - 4: Store the data in the file
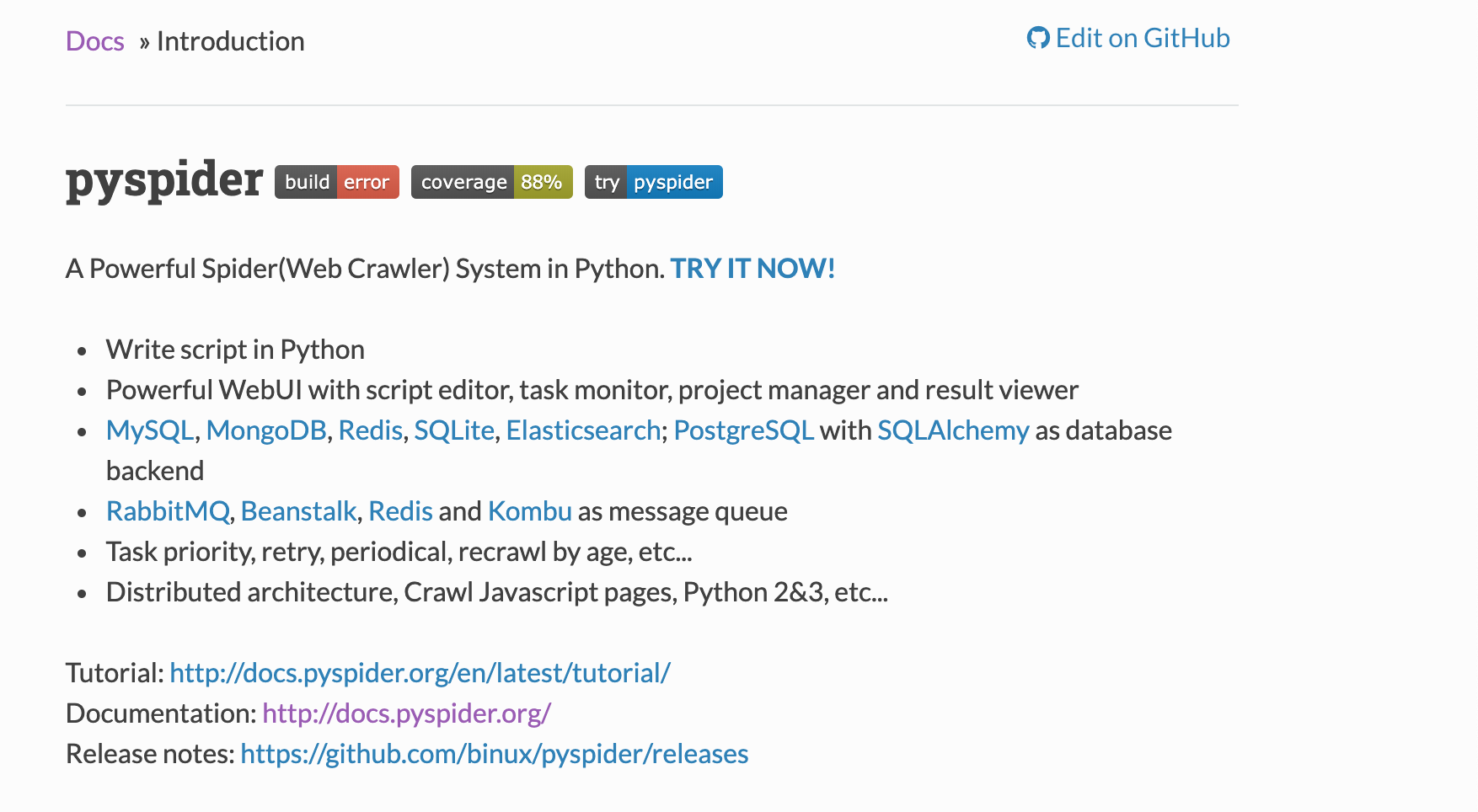
Store that information in required csv, xml, JSON file format.
Getting Started with Web Scrapping
Python has a vast collection of libraries and also provides a very useful library for web scrapping. Let's understand the required library for Python.
Library used for web scrapping
- Selenium- Selenium is an open-source automated testing library. It is used to check browser activities. To install this library, type the following command in your terminal.
Note - It is good to use the PyCharm IDE.
- Pandas
Pandas library is used for data manipulation and analysis. It is used to extract the data and store it in the desired format.
- BeautifulSoup
Let's understand the BeautifulSoup library in detail.
Installation of BeautifulSoup
You can install BeautifulSoup by typing the following command:
Installing a parser
BeautifulSoup supports HTML parser and several third-party Python parsers. You can install any of them according to your dependency. The list of BeautifulSoup's parsers is the following:
Parser | Typical usage |
---|---|
Python's html.parser | BeautifulSoup(markup,'html.parser') |
lxml's HTML parser | BeautifulSoup(markup,'lxml') |
lxml's XML parser | BeautifulSoup(markup,'lxml-xml') |
Html5lib | BeautifulSoup(markup,'html5lib') |
We recommend you to install html5lib parser because it is much suitable for the newer version of Python, or you can install lxml parser.
Type the following command in your terminal:
BeautifulSoup is used to transform a complex HTML document into a complex tree of Python objects. But there are a few essential types object which are mostly used:
- Tag
A Tag object corresponds to an XML or HTML original document.
Output:
Tag contains lot of attributes and methods, but most important features of a tag are name and attribute.
- Name
Every tag has a name, accessible as .name:
- Attributes
A tag may have any number of attributes. The tag <b id = 'boldest'> has an attribute 'id' whose value is 'boldest'. We can access a tag's attributes by treating the tag as dictionary.
We can add, remove, and modify a tag's attributes. It can be done by using tag as dictionary.
- Multi-valued Attributes
In HTML5, there are some attributes that can have multiple values. The class (consists more than one css) is the most common multivalued attributes. Other attributes are rel, rev, accept-charset, headers, and accesskey.
- NavigableString
A string in BeautifulSoup refers text within a tag. BeautifulSoup uses the NavigableString class to contain these bits of text.
Python Web Scraping Tutorial
A string is immutable means it can't be edited. But it can be replaced with another string using replace_with().
In some cases, if you want to use a NavigableString outside the BeautifulSoup, the unicode() helps it to turn into normal Python Unicode string.
- BeautifulSoup object
The BeautifulSoup object represents the complete parsed document as a whole. In many cases, we can use it as a Tag object. It means it supports most of the methods described in navigating the tree and searching the tree.
Output:
Web Scrapping Example:
Let's take an example to understand the scrapping practically by extracting the data from the webpage and inspecting the whole page.
First, open your favorite page on Wikipedia and inspect the whole page, and before extracting data from the webpage, you should ensure your requirement. Consider the following code:
Output:
In the following lines of code, we are extracting all headings of a webpage by class name. Here front-end knowledge plays an essential role in inspecting the webpage.
Output:
In the above code, we imported the bs4 and requested the library. In the third line, we created a res object to send a request to the webpage. As you can observe that we have extracted all heading from the webpage.
Webpage of Wikipedia Learning
Let's understand another example; we will make a GET request to the URL and create a parse Tree object (soup) with the use of BeautifulSoup and Python built-in 'html5lib' parser.
Here we will scrap the webpage of given link (https://www.javatpoint.com/). Consider the following code:
The above code will display the all html code of javatpoint homepage.
Using the BeautifulSoup object, i.e. soup, we can collect the required data table. Let's print some interesting information using the soup object:
- Let's print the title of the web page.
Output: It will give an output as follow:
- In the above output, the HTML tag is included with the title. If you want text without tag, you can use the following code:
Python Web Scraping Library
Output: It will give an output as follow:
- We can get the entire link on the page along with its attributes, such as href, title, and its inner Text. Consider the following code:
Output: It will print all links along with its attributes. Here we display a few of them:
Demo: Scraping Data from Flipkart Website
In this example, we will scrap the mobile phone prices, ratings, and model name from Flipkart, which is one of the popular e-commerce websites. Following are the prerequisites to accomplish this task:
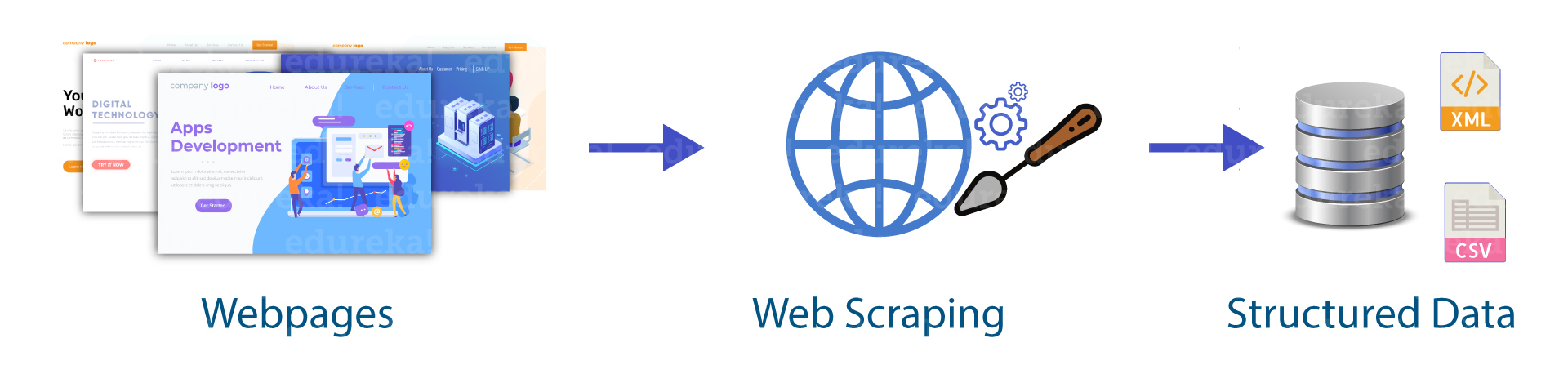
Prerequisites:
- Python 2.x or Python 3.x with Selenium, BeautifulSoup, Pandas libraries installed.
- Google - chrome browser
- Scrapping Parser such as html.parser, xlml, etc.
Step - 1: Find the desired URL to scrap
The initial step is to find the URL that you want to scrap. Here we are extracting mobile phone details from the flipkart. The URL of this page is https://www.flipkart.com/search?q=iphones&otracker=search&otracker1=search&marketplace=FLIPKART&as-show=on&as=off.
Step -2: Inspecting the page
It is necessary to inspect the page carefully because the data is usually contained within the tags. So we need to inspect to select the desired tag. To inspect the page, right-click on the element and click 'inspect'.
Step - 3: Find the data for extracting
Extract the Price, Name, and Rating, which are contained in the 'div' tag, respectively.
Step - 4: Write the Code
Output:
We scrapped the details of the iPhone and saved those details in the CSV file as you can see in the output. In the above code, we put a comment on the few lines of code for testing purpose. You can remove those comments and observe the output.
In this tutorial, we have discussed all basic concepts of web scrapping and described the sample scrapping from the leading online ecommerce site flipkart.
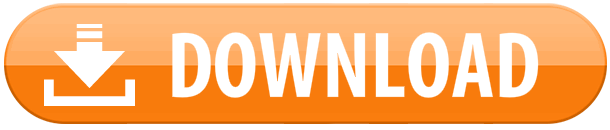